CEBRA
Learnable latent embeddings for joint behavioural and neural analysis
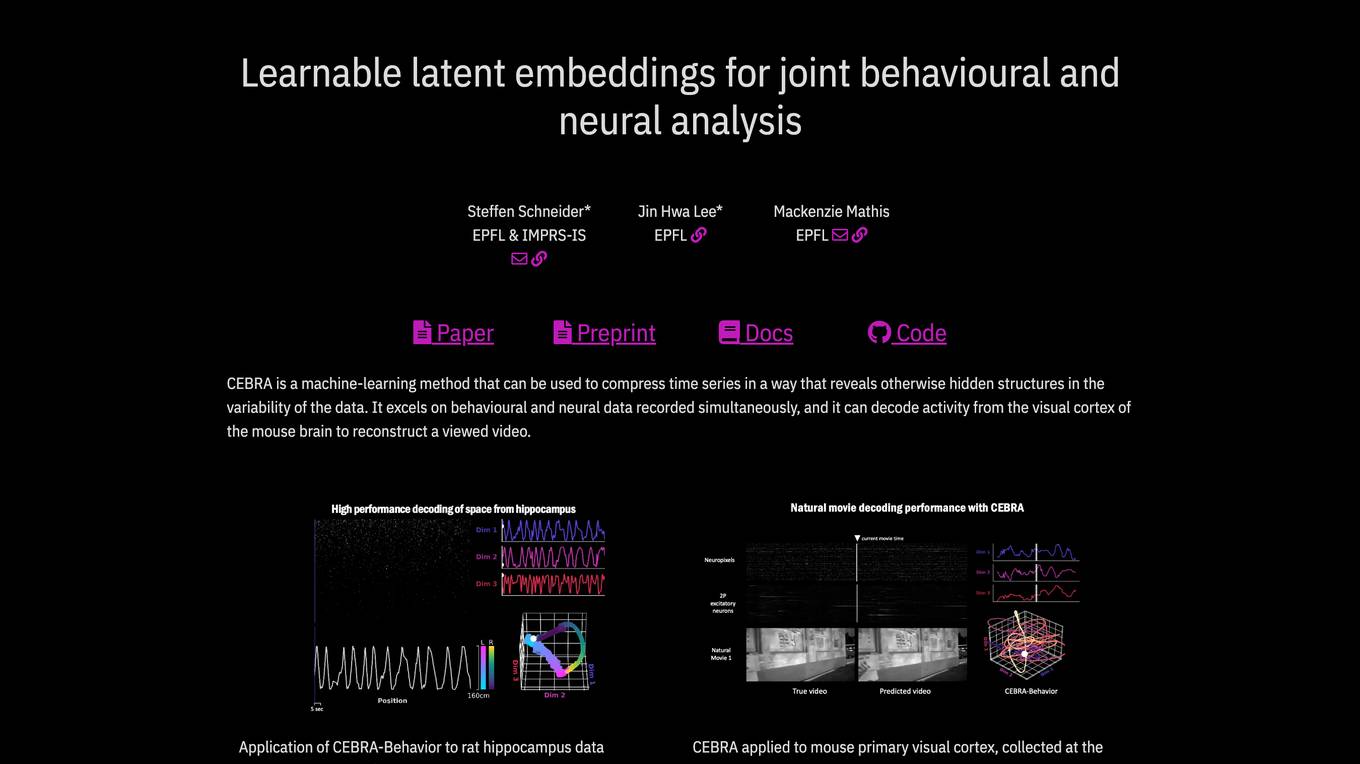
Description:
CEBRA (CompressEd Behavioural and Neural Analysis) is a machine-learning method that can be used to compress time series in a way that reveals otherwise hidden structures in the variability of the data. It excels on behavioural and neural data recorded simultaneously, and it can decode activity from the visual cortex of the mouse brain to reconstruct a viewed video.
CEBRA is a novel encoding method that jointly uses behavioural and neural data in a (supervised) hypothesis- or (self-supervised) discovery-driven manner to produce both consistent and high-performance latent spaces. We show that consistency can be used as a metric for uncovering meaningful differences, and the inferred latents can be used for decoding. We validate its accuracy and demonstrate our tool's utility for both calcium and electrophysiology datasets, across sensory and motor tasks, and in simple or complex behaviors across species. It allows for single and multi-session datasets to be leveraged for hypothesis testing or can be used label-free. Lastly, we show that CEBRA can be used for the mapping of space, uncovering complex kinematic features, produces consistent latent spaces across 2-photon and Neuropixels data, and can provide rapid, high-accuracy decoding of natural movies from visual cortex.
For Tasks:
For Jobs:
Features
- Can be used to compress time series in a way that reveals otherwise hidden structures in the variability of the data.
- Excels on behavioural and neural data recorded simultaneously.
- Can decode activity from the visual cortex of the mouse brain to reconstruct a viewed video.
- Can be used to map space, uncovering complex kinematic features.
- Produces consistent latent spaces across 2-photon and Neuropixels data.
- Can provide rapid, high-accuracy decoding of natural movies from visual cortex.
Advantages
- Can be used to uncover meaningful differences in data.
- Can be used for decoding.
- Has been validated on a variety of datasets, including calcium and electrophysiology datasets, across sensory and motor tasks, and in simple or complex behaviors across species.
- Can be used for hypothesis testing or can be used label-free.
- Is open-source and available on GitHub.
Disadvantages
- Can be computationally expensive to run.
- May not be suitable for all types of data.
- Requires a certain level of expertise to use.
Frequently Asked Questions
-
Q:What is CEBRA?
A:CEBRA is a machine-learning method that can be used to compress time series in a way that reveals otherwise hidden structures in the variability of the data. -
Q:What are the advantages of using CEBRA?
A:CEBRA can be used to uncover meaningful differences in data, can be used for decoding, has been validated on a variety of datasets, can be used for hypothesis testing or can be used label-free, and is open-source and available on GitHub. -
Q:What are the disadvantages of using CEBRA?
A:CEBRA can be computationally expensive to run, may not be suitable for all types of data, and requires a certain level of expertise to use.
Alternative AI tools for CEBRA
Similar sites
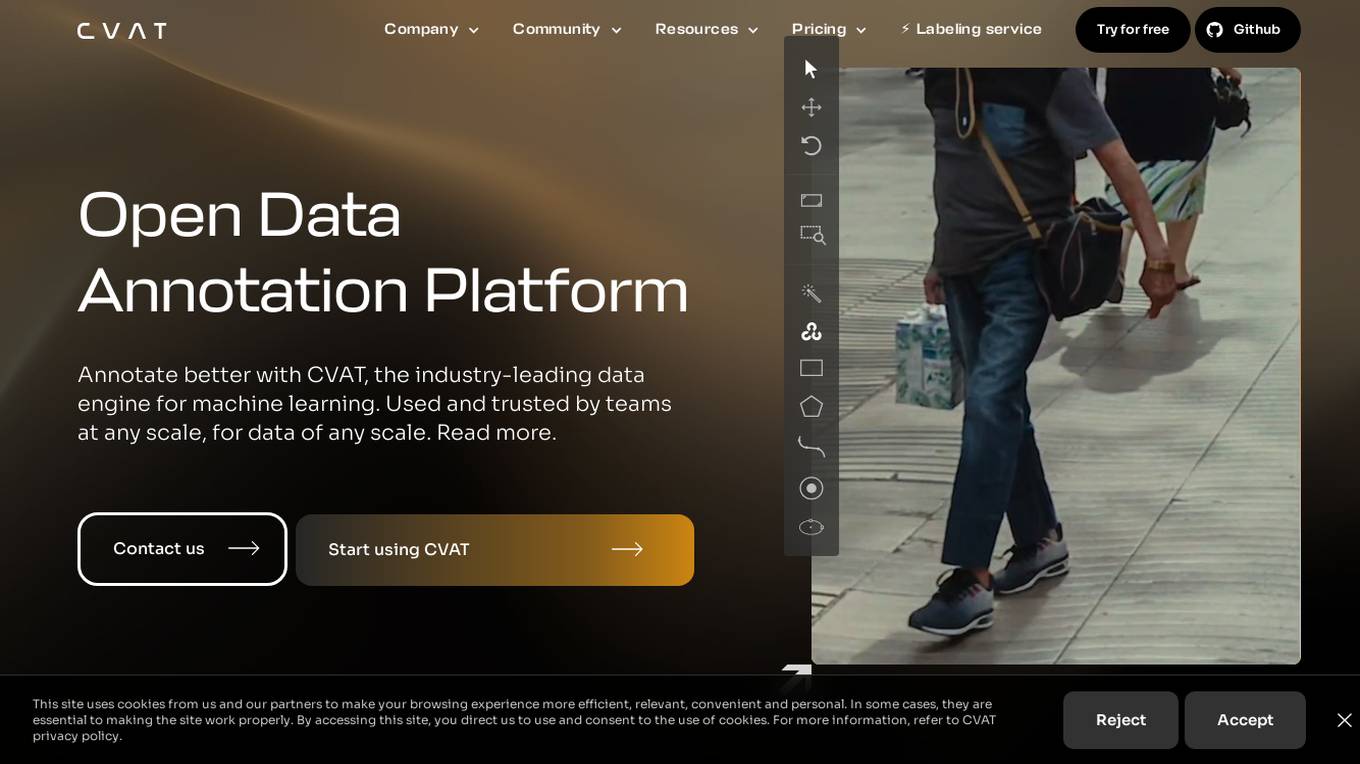
CVAT
Annotate better with CVAT, the industry-leading data engine for machine learning.
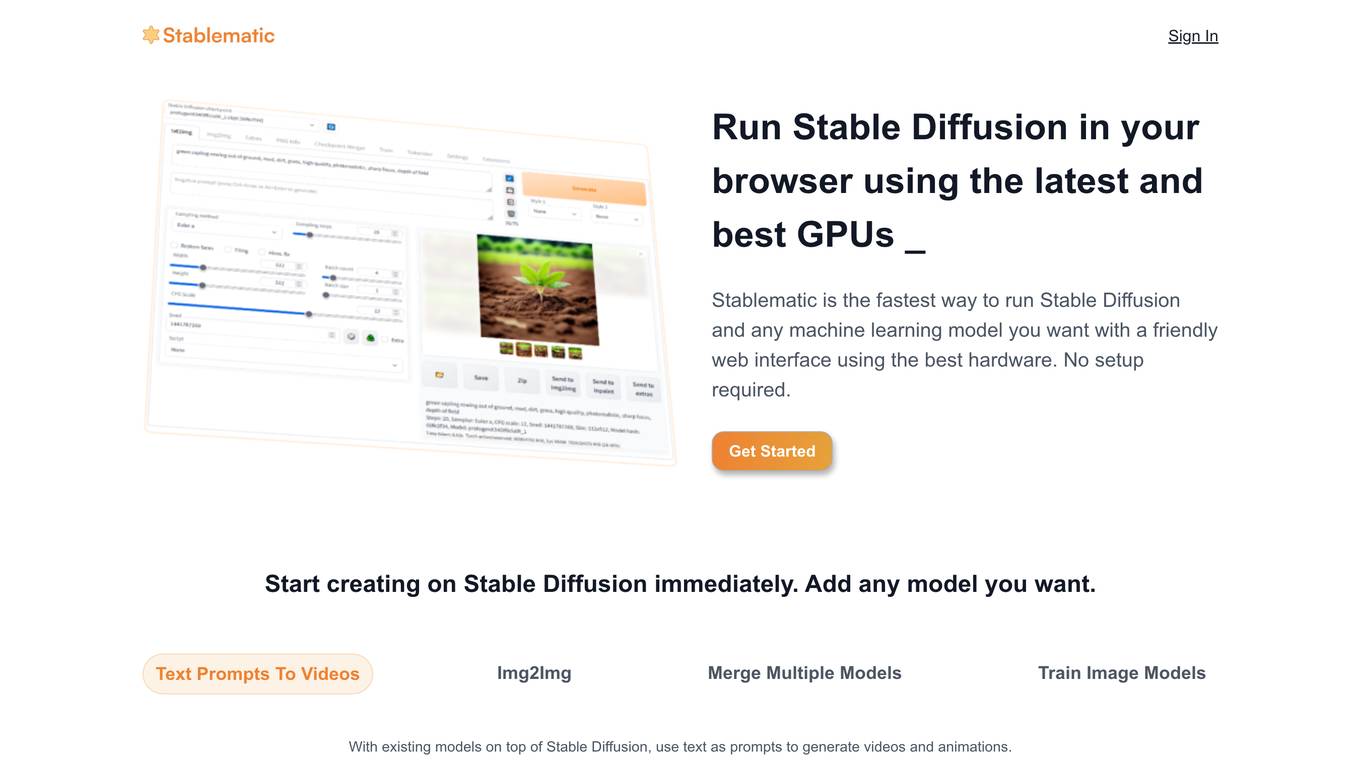
Stablematic
The fastest way to run Stable Diffusion and any machine learning model you want with a friendly web interface using the best hardware.
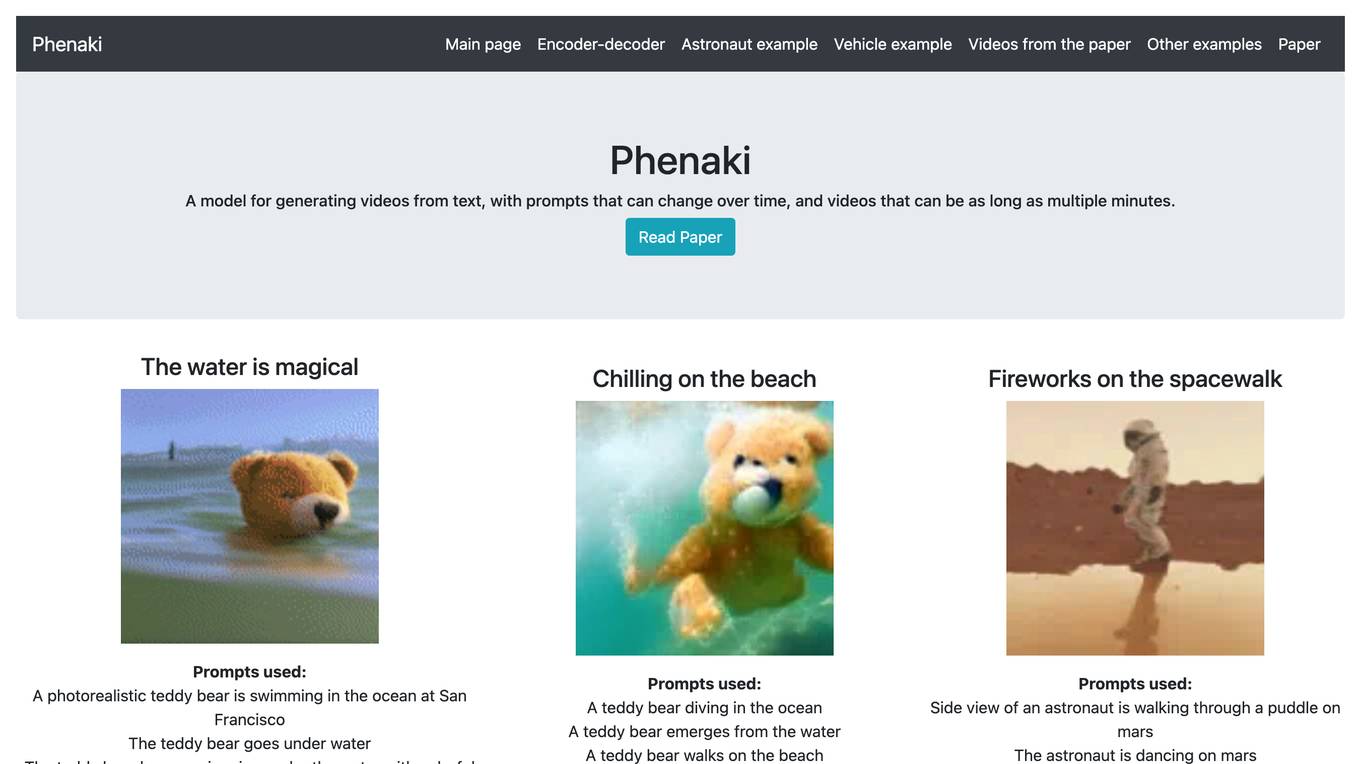
Phenaki
A model for generating videos from text, with prompts that can change over time, and videos that can be as long as multiple minutes.
For similar jobs
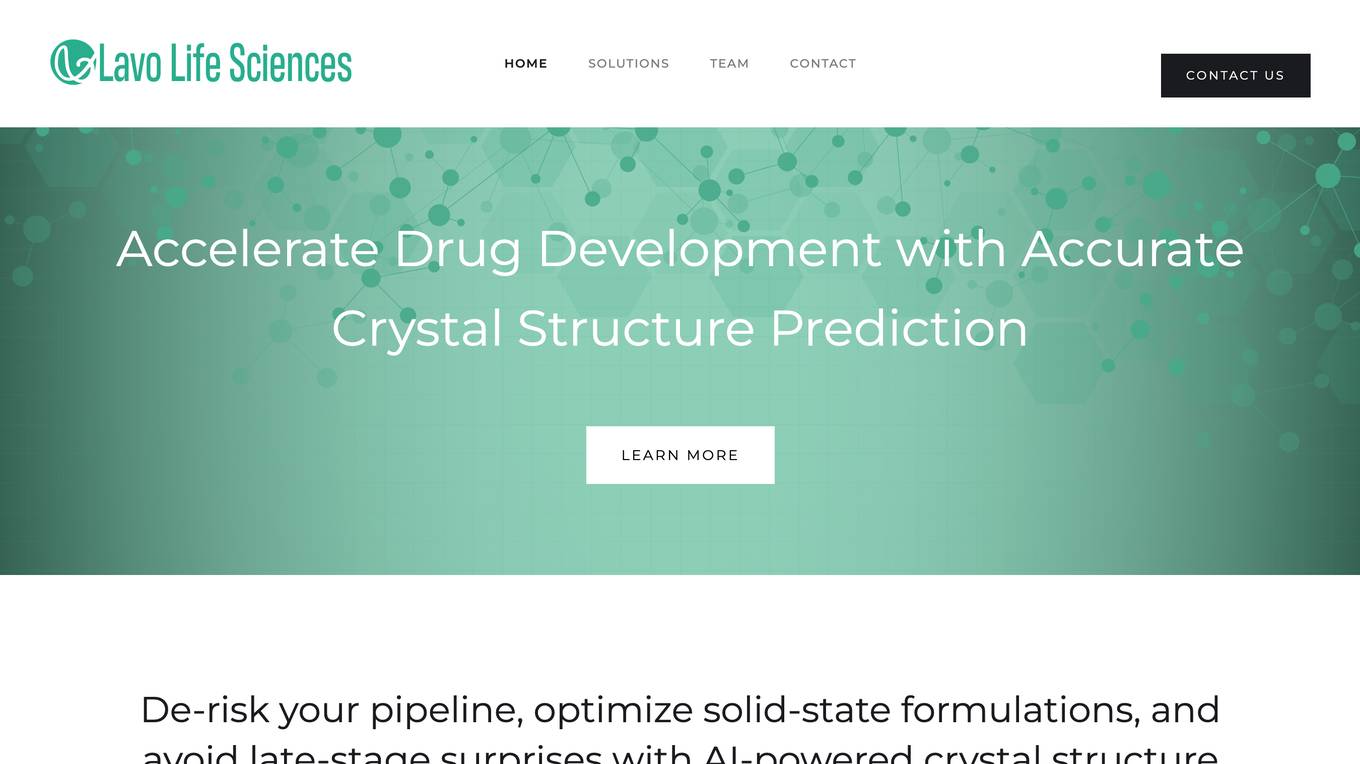
Lavo Life Sciences
Accelerate Drug Development with Accurate Crystal Structure Prediction